Last week I have received a comment on Stack Overflow on my answer to the question Fastest way to convert an iterator to a list that was asking if I could post some test results. The question has more than 100k views but there are no numbers regarding the fastest way to convert an iterator to a list so I decided to run some tests.
There are at least 3 ways to convert an iterator to a list:
1) by type constructor
list(my_iterator)
2) by unpacking
[*my_iterator]
3) using list comprehension
[e for e in my_iterator]
What is an iterator?
An iterator object conforms to the iterator protocol this means that will implement 2 magic methods:
The __iter__ will return the iterator object
The __next__ method will return the next value, this method will raise StopIteration exception when the iterator is exhausted, there are no more values to return.
Here is an example of an iterator that returns the first 10 integers:
class FirstTen:
def __init__(self):
self.current_value = 0
def __iter__(self):
return self
def __next__(self):
if self.current_value == 10:
raise StopIteration
result = self.current_value
self.current_value += 1
return result
Simple benchmark
In the following benchmark, I have used Python3.8 and the library simple_benchmark:
from simple_benchmark import BenchmarkBuilder
from heapq import nsmallest
b = BenchmarkBuilder()
@b.add_function()
def convert_by_type_constructor(size):
list(iter(range(size)))
@b.add_function()
def convert_by_list_comprehension(size):
[e for e in iter(range(size))]
@b.add_function()
def convert_by_unpacking(size):
[*iter(range(size))]
@b.add_arguments('Convert an iterator to a list')
def argument_provider():
for exp in range(2, 22):
size = 2**exp
yield size, size
r = b.run()
r.plot()
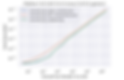
As you can see there is very hard to make a difference between conversion by the constructor and conversion by unpacking, conversion by list comprehension is the “slowest” approach.
Compare between different python versions
I have been comparing python 3.6, 3.7, 3.8, and the latest released version python 3.9.
To get the performance numbers and still keep it simple (no extra tools) I had to install all these versions. I had made the following simple script to get the comparison numbers:
import argparse
import timeit
parser = argparse.ArgumentParser(
description='Test convert iterator to list')
parser.add_argument(
'--size', help='The number of elements from iterator')
args = parser.parse_args()
size = int(args.size)
repeat_number = 10000
# do not wait too much if the size is too big
if size > 10000:
repeat_number = 100
def test_convert_by_type_constructor():
list(iter(range(size)))
def test_convert_by_list_comprehension():
[e for e in iter(range(size))]
def test_convert_by_unpacking():
[*iter(range(size))]
def get_avg_time_in_ms(func):
avg_time = timeit.timeit(func, number=repeat_number) * 1000 / repeat_number
return round(avg_time, 6)
funcs = [test_convert_by_type_constructor,
test_convert_by_unpacking, test_convert_by_list_comprehension]
print(*map(get_avg_time_in_ms, funcs))
The script will be executed via a subprocess from a Jupyter Notebook (or a script), the size parameter will be passed through command-line arguments and the script results will be taken from standard output.
Observation!
I have used the built-in function iter to convert to an iterator the built-in function range (which is not an iterator).
from subprocess import PIPE, run
import seaborn as sns
import pandas
simple_data = {'constructor': [], 'unpacking': [], 'comprehension': [],
'size': [], 'python version': []}
data = {'conversion type': [], 'timing in ms': [], 'size': [], 'python version': []}
size_test = 100, 1000, 10_000, 100_000, 1_000_000
for version in ['3.6', '3.7', '3.8', '3.9']:
print('test for python', version)
for size in size_test:
command = [f'python{version}', 'perf_test_convert_iterator.py', f'--size={size}']
result = run(command, stdout=PIPE, stderr=PIPE, universal_newlines=True)
constructor, unpacking, comprehension = result.stdout.split()
data['conversion type'].extend(['constructor', 'unpacking', 'comprehension'])
data['timing in ms'].extend([float(constructor), float(unpacking), float(comprehension)])
data['python version'].extend([version] * 3) # same version for each conversion type
data['size'].extend([size] * 3) # same size for each conversion type
simple_data['constructor'].append(float(constructor))
simple_data['unpacking'].append(float(unpacking))
simple_data['comprehension'].append(float(comprehension))
simple_data['python version'].append(version)
simple_data['size'].append(size)
I have 2 types of data, one to be more simple to plot through Pandas (simple_data) and one to plot grouped bar plots with seaborn (data),
df_ = pandas.DataFrame(simple_data)
df_

In most of the cases, in my tests, unpacking shows to be faster, but the difference is so small that the results may change from a run to the other. Again, the comprehension approach is the slowest, in fact, the other 2 methods are up to ~ 60% faster.
Here are a few plots from the obtained data to get a better understanding:
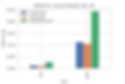
The following 2 plots represent the data obtained for the conversion by type constructor:


You can get the full notebook from here.
In conclusion, you should avoid list comprehension when you convert an iterator to a list, and from a performance viewpoint, you may get the same benefit if you use unpacking or type constructor.
Since we have 2 winners for the fastest conversion I will suggest to choose the conversion by type constructor:
list(my_iterator)
because is close to natural language making you 'instantly' think what is the output having a huge plus for readability.